Training for decision aid users focuses on making the aid
work, that is, on how to input required information and how
to change modes of aid operation. To benefit from a decision
aid, however, the user must learn to assess and act on uncertainty
about the quality of the aid's recommendations. Very little
attention has been paid to this. Yet very often acceptance
and/or effective use of a decision aid hinges on the need
by users to evaluate the aid's performance in real-time and
adjust their reliance on the aid in the light of that evaluation.
In research sponsored by the Aviation Applied Technology
Directorate, ATCOM, Fort Eustis, VA, CTI developed a systematic
framework for training users of decision aids in the appropriate
level of trust. We applied and tested the framework by developing
a training strategy for a specific decision aiding environment,
the Rotorcraft Pilot's Associate.
The first step was the development of a model of a user's
trust in a decision aid. The Situation Specific
Trust model is intended to account for the basic findings
of recent empirical research on trust in automation, and extend
those principles to decision aids. The model has two complementary
aspects:
- A qualitative aspect, based on the structure of arguments
about expected system performance.
- A quantitative aspect, based on probability
event trees.
Trust as an Argument about Aid Performance |
The qualitative part of the model treats trust as a
reasoned conclusion about the likelihood of good or
bad aid performance in a particular situation. In short,
trust represents an dynamically updated argument. Following
Toulmin's notion of argument structure, we identify
five components of trust (as shown in the figure below):
- Grounds = awareness of current features
of the system and the situation.
- Claim = (the probability of) correct
system action over a specified period of time t conditional
on the observed features.
- Qualification of the claim = the probability,
or other less formal expression of uncertainty, assigned
to the claim.
- Warrant = the belief that certain
features of the system and situation are in general
correlated with system performance.
- Backing = the type of source of the
warrant, for example, the user's experience with the
system, knowledge of system design, or assumptions.
|
The model also identifies parameters of variation in these
trust components:
- Completeness of the features recognized
in the grounds and warrant.
- Reliabilty of the information used for backing.
- Temporal scope of the claim.
- Resolution and calibration
of the probabilistic qualification of the claim.
If you pass your mouse over figure above, you will see descriptions
of the parameters of each component.
Trust as Expected Value in a Probabilistic
Event Tree |
The quantitative aspect of the Situation Specific Trust
model represents trust as a dynamically evolving probability,
or expected value, within an event tree
(illustrated in the figure below).
The event tree represents all relevant situations for
aid use that have been discriminated by the user. A
single path through the tree represents
the user's experience of acquiring new information about
the system and the environment over time in a particular
context.
Both the components and parameters of arguments about
trust can be clarified and made more rigorous by reference
to aspects of such event trees.
|
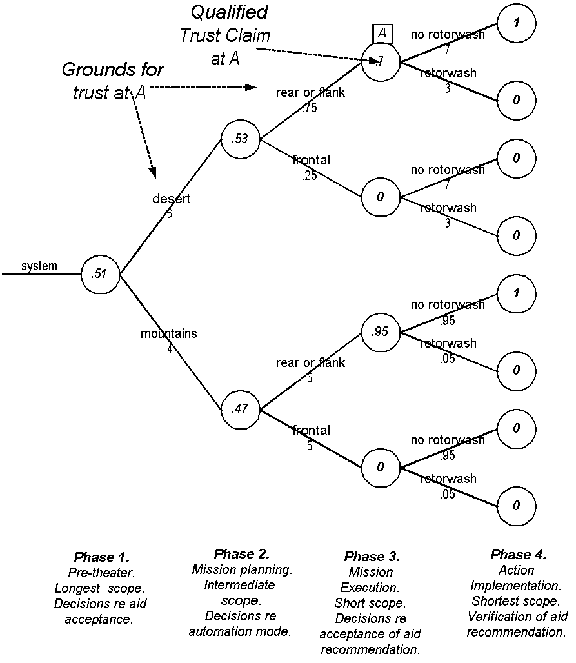
User Reliance Decisions |
A final part of the trust model pertains to user-decision
aid interaction. The model clarifies the
strategies available to decision aid designers and users
at different phases in the career of the decision aid.
For example:
- Decisions about system automation capabilities are
made at the design stage.
- Users or managers select the desired automation
mode and adjust aid parameters during the planning
of an operation.
- Users choose either to comply or not comply with
an aid's recommendations during execution of the operation.
By incorporating these decisions into the event tree
at the appropriate points, methods for evaluating interaction
options can be specified.
|
The following diagram illustrates a simple algorithm for
deciding whether to accept, reject, or think more about an
aid recommendation. The decision is a function of three variables:
- trust in the aid
- cost of delay
- the cost of errors
Each variable is likely to change as time passes. The curving
dashed line, for example, represents the evolution of trust
in the aid as a function of time and new information. The
envelop defined by the two dotted lines is a function of the
cost of delay and the cost of errors, and defines the region
in which critical thinking about an aid recommendation is
worthwhile, rather than immediate acceptance or rejection.
At the start (far left), trust is not high enough to accept
the aid recommendation and not low enough to reject it under
the prevailing conditions of the other two variables. As time
passes, trust increases somewhat, while the window for critical
thinking about the aid's recommendation narrows, e.g., because
the marginal cost of further delay increases with the length
of the delay. In this example, after critical thinking to
verify the aid's advice, the level of trust crosses the upper
boundary, leading the user to accept the aid recommendation.
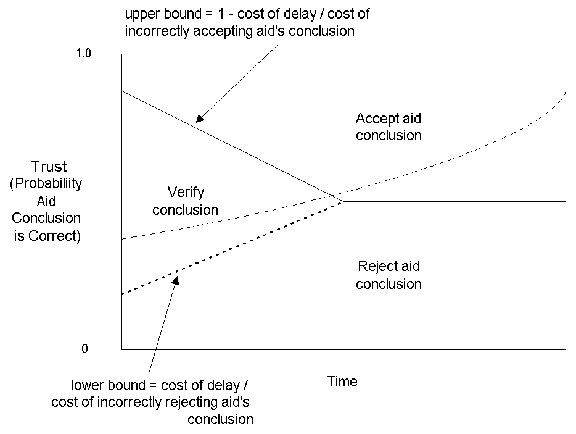
The algorithm used to generate this figure reflects the same
principles embodied in the Quick Test in the Recognition-Metacognition
model.
The Situation-Specific Trust model provides an account of
the mental models required
by effective decision aid users at each phase of decision
aid use, and the monitoring and situation awareness skills
required to use those mental models effectively. In addition,
the probabilistic event tree representation is a tool for
generating training scenarios in which users can acquire the
relevant mental models. The event tree also provides diagnostic
measures to assess the progress of trainees in learning to
use the aid and the effectiveness of the training.
The Situation Specific Trust model was tested by employing
it to design a demonstration training strategy for the Combat
Battle Position Planner module of the Rotorcraft Pilot's Associate
(RPA). The training strategy has two main parts:
- It conveys a mental model of situation and system features
that are correlated with good and bad aid performance
- It introduces users to specific user-aid interaction strategies
that are appropriate under different circumstances.
The illustrative training package was evaluated by four experienced
pilots. In their comments, the pilots emphasized two results
of the training: (1) acquiring increased understanding of
the Combat Battle Position Planner and (2) learning new ways
to interact with it. These findings lay the groundwork for
more extensive RPA training that is being developed in the
present phase the project.
See also:
|